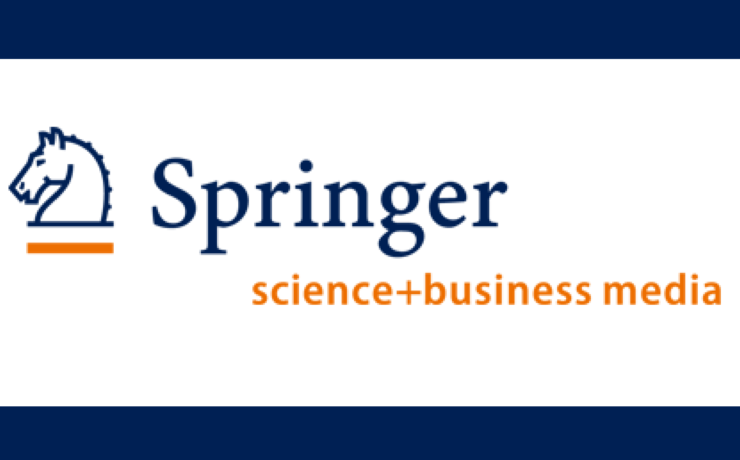
Image quality of ultralow-dose chest CT using deep learning…
Objective: To compare the image quality between the vendor-agnostic and vendor-specific algorithms on ultralow-dose chest CT.
Objective: To compare the image quality between the vendor-agnostic and vendor-specific algorithms on ultralow-dose chest CT.
To evaluate the image quality of low iodine concentration, dual-energy CT (DECT) combined with a deep learning–based noise reduction technique for pediatric abdominal CT, compared with standard iodine concentration single-energy polychromatic CT (SECT).
To assess the feasibility of applying a deep learning-based denoising technique to coronary CT angiography (CCTA) along with iterative reconstruction for additional noise reduction.
This study determined the effect of dose reduction and kernel selection on quantifying emphysema using low-dose computed tomography (LDCT) and evaluated the efficiency of a deep learning–based kernel conversion technique in normalizing kernels for emphysema quantification.
To examine the potential effect of CT dose variation on radiomic features in vivo using simulated contrast-enhanced CT dose reduction in patients with non-small lung cell cancer (NSCLC).
To compare image qualities between vendor-neutral and vendor-specific hybrid iterative reconstruction (IR) techniques for abdominopelvic computed tomography (CT) in young patients.
To determine the effect of a vendor unspecific, DICOM-based denoising technique on image quality and diagnostic performance in low-dose simulated abdominal computed tomography (CT) examinations in patients with suspected appendicitis.
To examine the impact of denoising on ultra-low-dose volume perfusion CT (ULD-VPCT) imaging in acute stroke.